AI Customer Segmentation: Identifying High-Value Customer Groups
- Danny Mukiza
- Sep 11, 2024
- 8 min read
Updated: Sep 28, 2024
Intro: What is AI Customer Segmentation?
Customer segmentation is the process of dividing a customer base into distinct groups based on shared characteristics. These characteristics range from demographic data, age and location, to behavioral data like purchasing frequency or brand engagement.
Remember the old days of customer segmentation? You’d group people by age, location, and maybe their last purchase, hoping for the best.
That was great for a start, but let’s face it—customer behavior is anything but static, especially in 2024. With customer sentiment & buying behaviour changing as fast as the latest social media trend, how do you keep up?
Well, that’s where AI-driven customer segmentation comes in.
Artificial intelligence revolutionizes customer segmentation by enabling businesses to dynamically adapt their segments in real-time, using vast amounts of customer data.
This approach allows businesses to target these groups with personalized marketing efforts, resulting in higher engagement and stronger returns on investment (ROI).
The importance of finding high-value customer groups cannot be overstated. These are the customers who typically represent the most loyal, engaged, and profitable segments of a business’s audience. The VIP's.
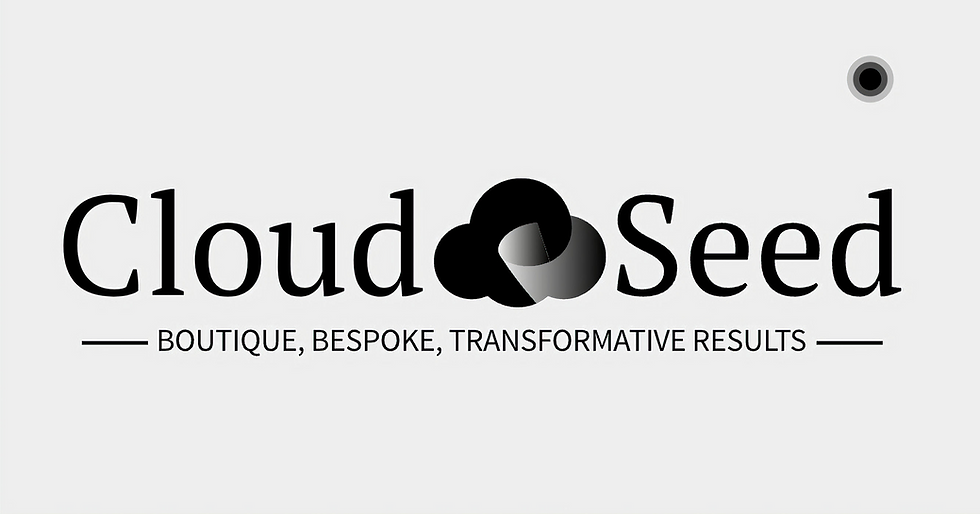
Table Of Contents - (So you can jump around 😉)
Traditional vs. AI-Driven Segmentation Models: Whats the Difference?
Traditional segmentation methods, which often rely on broad categories, usually miss the nuances of customer behavior, especially in fast-moving digital environments like eCommerce & DTC
Unlike static, traditional segmentation methods, AI-driven customer segmentation analyzes patterns, predicts future behaviors, and identifies the most valuable customer groups based on predictive analytics and historical data.
Traditional Segmentation Methods: A Deeper Look
Traditional segmentation techniques typically involve grouping customers based on static attributes, demographics (age, income, gender), geography (location, region), or psychographics (lifestyle, interests).
While still valuable, these approaces are limited in their ability to adapt to fast-changing and dynamic customer behaviors.
Plus, they require manual updates and time-consuming data analysis, making it challenging for businesses to keep up with rapidly changing customer preferences.
AI-Driven Segmentation: The Dynamic Powerhouse

AI, on the other hand, transforms customer segmentation into a dynamic, data-driven evolving process.
AI-powered models use techniques like clustering and classification models, to analyze customer behaviors, purchase history, and engagement patterns as they happen.
This lets businesses continuously refine their customer segments and adjust their marketing efforts accordingly.
For instance, AI might spot that a customer who usually buys low-ticket items suddenly starts browsing premium products. The algorithm updates their segment instantly, making sure your marketing stays as agile as your customers.
Predictive Analytics for High-Value Customer Identification
One of the most powerful applications of AI solutions in customer segmentation is predictive analytics, which uses historical data to forecast future customer behaviors.
By identifying customers who are likely to become high-value VIPs, businesses can focus their marketing and retention efforts & dollars on these customers since they'd be the most likely to contribute to long-term profitability.
Say goodbye to inefficient marketing spend.
Finding High-Value Customers Using AI
Predictive analytics enables businesses and DTC brands to more accurately identify their high-value customers & with that, calculate a more precise Customer Lifetime Value (CLV), a key metric that estimates the total revenue a customer will generate over their lifetime with the brand.
By using AI algorithms to analyze purchase history, frequency of interactions, and engagement metrics, brands can predict more accurate CLV numbers.
This enhanced precision allows businesses to tailor digital marketing strategies & deploy their marketing dollars more efficiently, allowing them to maximize the value of these high-CLV customers through personalized offers, loyalty programs, and tailored promotions.
You can learn more about AI-powered personalized offers & content in our guide.
Case Study
For example, an e-commerce brand using predictive analytics can find a segment of customers likely to make high-value purchases during specific seasons, like the holidays, BFCM, and other stunning insights.
By recognizing this pattern early, the brand can ready its inventory and marketing campaigns to target these customers with personalized recommendations, driving increased sales during peak periods.
AI Segmentation Techniques: Clustering and Classification Models

Clustering Techniques
AI-powered clustering models group customers based on shared characteristics. There are few different alogorityhmic methods businesses can use to do this.
K-means clustering: Divides customers into k clusters, where each customer belongs to the cluster with the nearest mean.
Hierarchical clustering: Which, creates a tree-like hierarchy of clusters, either through agglomerative (bottom-up) or divisive (top-down) approaches.
DBSCAN: Density-based clustering that groups together points that are closely packed together.
For instance, K-means clustering or hierarchical clustering algorithms group customers by factors like purchasing power, frequency of engagement, and the types of products purchased. It puts them in groups (clusters) with other customers that share their traits.
These clusters help businesses understand which customers are driving the most revenue and loyalty.
Clustering reveals hidden customer segments and patterns in customer data that businesses may not have previously seen.
A retail brand might discover a cluster of “frequent buyers” who make lower-value purchases regularly but contribute tons to long-term revenue due to their high purchase frequency.
Classification Models
For segmentation, classification models use customer behavior and engagement data to predict the likelihood of a customer becoming high-value or churning.
These models categorize customers into predefined segments based on behaviors, helping businesses focus on deploying retention campaigns for customers at risk of leaving or up-selling to customers on the verge of upgrading to premium services.
Salesforce Einstein and Klaviyo are some helpful tools that come pre-built with these AI-powered solutions that enable businesses to automate these processes and provide real-time segmentation and action-based insights for more precise customer targeting.
What is Behavioral and Psychographic Segmentation?
While behavioral segmentation focuses on customer actions, like purchase frequency and browsing habits, psychographic segmentation dives deeper into customer motivations, values, and attitudes. Combining these two segmentation methods using AI provides an even more granular understanding of customer needs.
Behavioral Segmentation with AI
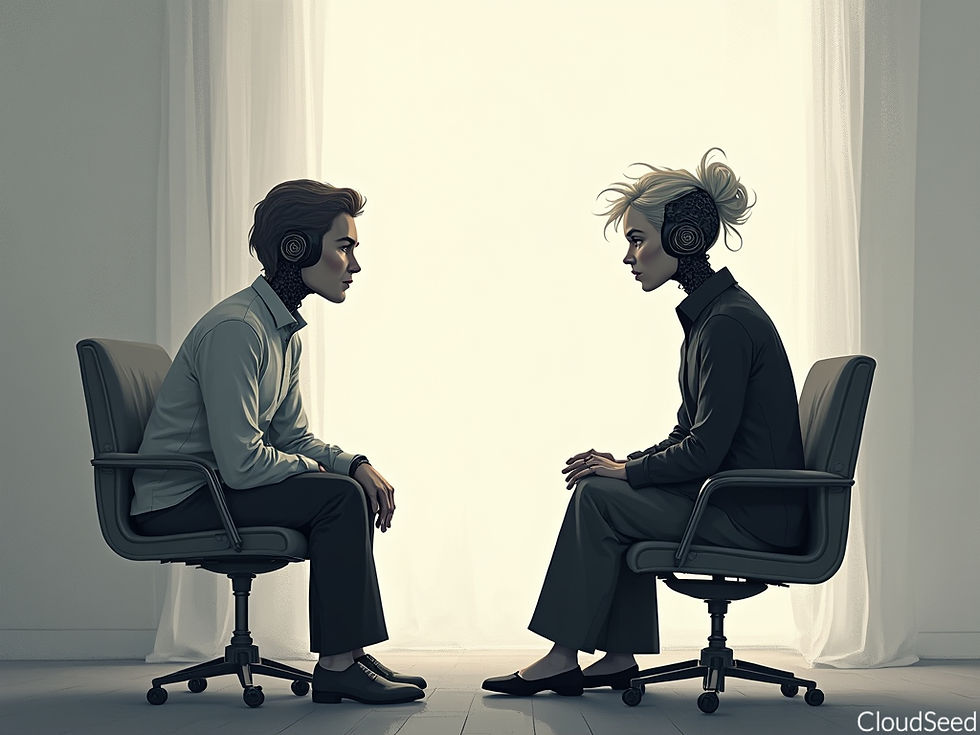
Behavioral segmentation involves analyzing a customer’s interaction with a brand across multiple channels, including website behavior, purchase patterns, and responses to marketing campaigns. AI enhances this by processing vast datasets and identifying trends that manual analysis might miss.
Psychographic Segmentation and NLP
AI’s natural language processing (NLP) abilities make psychographic segmentation even more powerful.
NLP allows AI to analyze unstructured data; customer reviews, social media posts, and feedback, to gain insights into customer sentiment and values.
This data helps e-commerce and DTC businesses create more emotionally resonant marketing campaigns that align with customer motivations.
Personalization With AI Segmentation
Personalized marketing is the likely future of customer engagement, and AI-driven segmentation enables businesses to tailor experiences on a micro level.
Personalizing customer interactions ensures that every interaction feels bespoke & personal, whether it’s a custom email offer, product recommendation, or loyalty perk.
Tailored Experiences
As an example, an ecom store can offer recommendations based on a customer’s browsing history, past purchases, and predicted future behaviors.
AI-powered segmentation makes it possible to create hyper-personalized experiences, meaning that each customer receives the right message, at the right time, through the right channel.
Data Quality and Privacy in AI Segmentation
AI offers powerful tools for segmentation, but the quality of the data that feeds these models is paramount.
Bad or poor data can lead to inaccurate predictions and flawed customer segments. Which will do more harm than good.
Data Integrity
AI models rely on clean, accurate data to be effective. Data cleansing processes, which remove duplicates, correct errors, and standardize formats, are essential to giving you a better chance that your AI-driven segmentation delivers reliable insights.
Compliance with Data Privacy Regulations
As businesses increasingly rely on customer data for segmentation, they must also navigate growing concerns over data privacy. Compliance with regulations like GDPR and CCPA is critical to maintaining customer trust.
Companies must implement robust security measures, including data encryption and access controls, to protect sensitive customer information .
Best Practices for AI Segmentation
Data Collection and Preparation
Effective AI segmentation starts with high-quality data as discussed above. Companies need to have a wide variety of customer data, ranging from purchase history to social media interactions, to feed into AI algorithms. The more data points you have the more insightful your insights.
Choosing the Right Tools
The AI tools used for segmentation should be scalable, flexible, and easily integrated into existing CRM systems. Platforms like Klaviyo and Segment provide a range of features for real-time segmentation and customer insights.
Ongoing Model Evaluation
Regular monitoring of AI models is essential to make sure they continue to deliver accurate and actionable insights. Feedback loops, where customer behavior informs future segmentation models, help companies stay agile and responsive.
Future Trends in AI-Driven Customer Segmentation
As AI technologies continue to evolve, new capabilities will surely come along, and advancements in predictive analytics and natural language processing will further enhance customer segmentation. These innovations allow businesses to anticipate customer needs more accurately, creating a deeper level of personalization that resonates with individual preferences.
Ethical Considerations
With AI’s increasing role in customer data analysis, businesses must remain mindful of ethical considerations, particularly in data privacy. Transparency and ethical data usage will be essential as AI segmentation becomes more sophisticated.
Final Thoughts
AI-driven customer segmentation is a game-changer for businesses looking to identify and engage their most valuable customer groups. By leveraging predictive analytics, real-time data processing, and personalized experiences, businesses can increase customer loyalty, reduce churn, and drive long-term profitability.
For businesses looking to stay ahead in the digital age, now is the time to explore AI solutions for their business, and implement these advanced segmentation strategies.
These technologies will help not only find but also fine-tune & curate experiences that resonate with your high-value customers and secure stronger engagement and higher ROI.
Fin.
Contact CloudSeed for a consultation today!
FAQ's
What is AI customer segmentation, and how is it different from traditional methods?
AI-driven customer segmentation uses machine learning and predictive analytics to analyze large datasets, such as customer behavior, purchasing history, and demographics. Unlike traditional segmentation, which relies on only static data (e.g., age, location), AI constantly updates segments in real-time, providing more dynamic and accurate insights. This allows for more personalized marketing strategies and the identification of high-value customer groups.
How does AI identify high-value customer segments?
AI analyzes historical and behavioral data using predictive analytics to calculate each customer’s Customer Lifetime Value (CLV). AI techniques like clustering and classification, segment customers based on their likelihood to spend more or become loyal. These insights allow businesses to focus their marketing efforts on high-value customers, such as frequent buyers or customers with higher purchase volumes.
What kind of data is needed for effective AI segmentation?
Effective AI segmentation requires data from multiple sources, such as:
• Purchase history (what they bought)
• Browsing behavior (what they are interested in)
• Engagement metrics (interactions with marketing campaigns)
Integrating this data allows AI to create dynamic, real-time customer profiles that evolve as your customers preferences change.
How to use AI in e-commerce to predict customer behavior?
Using predictive analytics, AI can scan historical customer data, identifying patterns that help forecast future behaviors. For example, AI can predict when customers are at risk of churn, which products they are likely to buy next, or which customers will have the highest CLV. Businesses can then tailor their marketing strategies accordingly.
How can AI improve personalized marketing?
AI can create hyper-personalized experiences by dynamically adjusting content, like email recommendations, product offers, and website content, based on individual customer segments. By using real-time data, AI ensures that marketing messages and offers are always relevant to a customer’s current needs and preferences.
What are the main AI techniques used in customer segmentation?
The key AI techniques for segmentation include:
• Clustering: Grouping customers based on similar behaviors, such as buying frequency or product preferences.
• Classification: Categorizing customers into predefined groups, such as high-value customers or potential churn risks.
• Predictive analytics: Using data to predict future customer actions, like repeat purchases or upgrades.
How to keep data private when using AI-driven segmentation?
Businesses must comply with privacy regulations such as GDPR and CCPA when using AI for customer segmentation. Ensuring data security involves using encryption, anonymization, and regular audits to protect customer data. AI can also be programmed to operate within these privacy frameworks, ensuring that sensitive customer information is not misused.
How can AI-driven customer segmentation drive eCommerce business growth?
By identifying high-value or high-churn-risk customer segments, AI-driven segmentation helps businesses target the right customers with personalized offers, improving customer retention and conversion rates. AI can help businesses uncover new opportunities for product or service innovation by analyzing customer feedback and behavior to drive long-term growth.
Comments